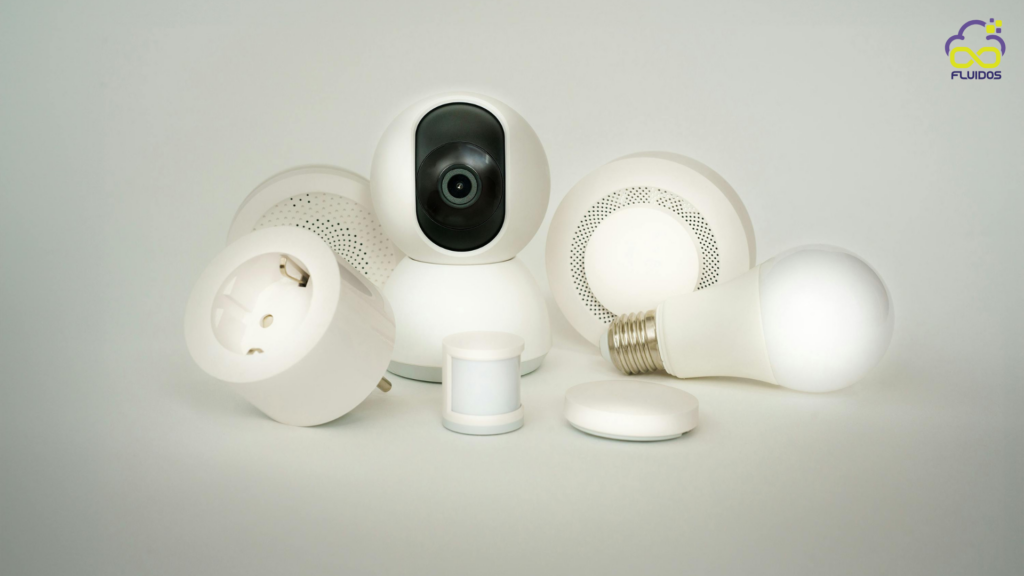
How FLUIDOS Enables Predictive Maintenance for EDGE Devices
In a world increasingly reliant on interconnected systems and smart infrastructure, predictive maintenance has emerged as one of the most impactful applications of the Internet of Things (IoT). The ability to anticipate failures before they occur not only reduces downtime and maintenance costs but also extends the life of critical equipment. However, achieving this at scale requires more than just smart sensors—it demands intelligent, decentralized orchestration. This is where FLUIDOS steps in.
What Is FLUIDOS?
FLUIDOS is an open-source, decentralized orchestration platform designed to optimize the performance of edge computing systems. By dynamically managing workloads, resources, and data flows across heterogeneous edge devices, FLUIDOS creates a more responsive, scalable, and cost-effective alternative to traditional cloud-based architectures.
But beyond orchestration, FLUIDOS provides a robust framework for enabling intelligent applications—predictive maintenance being a key one.
The Predictive Maintenance Challenge
Traditional predictive maintenance systems often rely on centralized cloud processing. Sensors collect data and send it to remote servers where machine learning models analyze it to detect anomalies or wear patterns. While effective in theory, this approach suffers from major drawbacks:
- High bandwidth and cloud costs
- Latency that can slow down real-time decision-making
- Reliance on connectivity in remote or high-risk environments
To address these limitations, FLUIDOS brings the intelligence closer to the devices, allowing predictive maintenance to happen on the edge.
How FLUIDOS Makes It Possible
- Local Data Processing and Filtering
FLUIDOS empowers edge nodes to pre-process sensor data locally. Instead of sending raw data to the cloud, edge devices can analyze vibration patterns, temperature spikes, or operational irregularities in real time. Only relevant insights or alerts are sent upstream, drastically reducing data volume and transmission costs.
- AI at the Edge
By distributing machine learning models across edge nodes, FLUIDOS allows for real-time anomaly detection directly at the source. These models can be trained centrally and deployed locally, ensuring that edge devices learn over time and improve their fault detection accuracy with minimal latency.
- Dynamic Resource Allocation
Predictive maintenance tasks often require more computing power than typical edge workloads. FLUIDOS dynamically orchestrates resources, ensuring that computationally intensive tasks like model inference are offloaded to more powerful nearby devices, if needed, without disrupting the overall system.
- Self-Healing and Resilience
In case of potential failures, FLUIDOS can proactively reroute tasks or alert maintenance personnel before a device fails completely. This self-healing capability increases the reliability of the system and prevents cascading failures in critical environments.
Real-World Impact: FLUIOT in Bangkok
One of the best demonstrations of FLUIDOS in action is the FLUIOT pilot deployment in Bangkok, where edge-enabled predictive maintenance was implemented across various urban infrastructure systems.
Key outcomes included:
- Reduction in unplanned outages by 30%
- Faster response times to detected anomalies
- Improved maintenance scheduling, reducing unnecessary interventions
By leveraging FLUIDOS, operators in Bangkok were able to extend the lifecycle of expensive infrastructure, save operational costs, and reduce their dependency on cloud-based analytics.
Towards a Smarter, More Resilient Edge
FLUIDOS doesn’t just support predictive maintenance—it redefines how it can be done. By turning the edge into an active participant in intelligent decision-making, FLUIDOS unlocks new possibilities for IoT systems that are faster, greener, and more autonomous.
Whether it’s deployed in smart cities, industrial environments, or remote energy installations, FLUIDOS lays the foundation for predictive maintenance that is scalable, cost-effective, and future-proof.
Disclaimer
The information reflects only the Author’s views and that the European Commission
cannot be found liable for any use that may be made of the information contained therein.