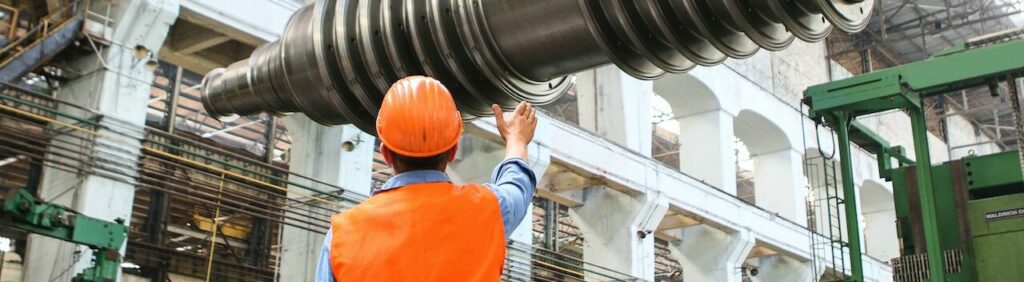
Tackling Quality Control Challenges in IoT Manufacturing
In the dynamic landscape of IoT manufacturing, the JitJip project takes a bold step to address common quality control challenges that have long plagued the industry. These challenges include:
- Verifying Device Components
One of the prominent hurdles in IoT manufacturing is ensuring that each device contains the correct sub-modules. IoT devices often come with modular designs that can incorporate additional functions based on customer requests. The verification process is vital to confirm that each device aligns with its intended specifications.
- Waterproofing Requirements
Waterproofing is of paramount importance for IoT devices, and the JitJip project is laser-focused on verifying that each device complies with the environmental requirements necessary for a waterproof case. Since connectors are susceptible to water leaks, ensuring waterproofing is essential to prolong device lifespan.
- Antenna Installation
In scenarios where a device’s enclosure is metallic, the project places special emphasis on guaranteeing the proper installation of external antennas. This precaution is crucial to prevent external antennas from inadvertently making contact with the metal casing, a common production error.
- Tracking Faults
Throughout its duration, the JitJip project will meticulously monitor faults and sensor return rates at each manufacturing site. This data will play a pivotal role in evaluating the effectiveness of AI algorithms by comparing fault rates before and after AI deployment.
- Generating Reports
As part of the project, regular quality management reports and recommendations will be crafted. This practice allows continuous quality management enhancement and optimization based on real-world data.
AI-Based Image Processing and Data Fusion
The pilot demonstration of the JitJip project centers on AI-powered image processing, enriched with data from various sources. This approach encompasses the creation of video libraries and neural network training, incorporating data from Correlation Systems’ existing sensor manufacturing processes and information from faulty sensors returned by customers or discovered during production. This data-driven strategy forms the foundation for potentially commercializing the JitJip system, which can be extended to manufacture other IoT devices from third-party providers.
In brief, the JitJip project serves as a beacon of innovation in the IoT manufacturing landscape, striving to conquer persistent quality control issues. By addressing these challenges and integrating AI, the project has the potential to elevate the industry, ensuring the production of more reliable and consistent IoT devices for the betterment of both manufacturers and end-user.
Disclaimer
The information reflects only the Author’s views and that the European Commission or KITT4SME
cannot be found liable for any use that may be made of the information contained therein.
